Harnessing the Power of Data: How Machine Learning is Revolutionizing Microgrid Energy Management
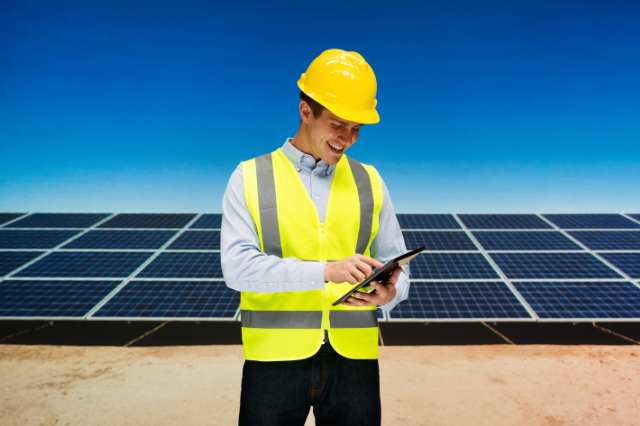
The use of machine learning and artificial intelligence (AI) in microgrid energy management has the potential to significantly improve the efficiency and reliability of energy systems, leading to cost savings, improved reliability, and more sustainable energy systems.
Machine learning has become popular in microgrid technology for several reasons:
The complexity of microgrids: Microgrids are complex systems that involve multiple energy sources, energy storage systems, and energy consumers. Machine learning algorithms are capable of handling large amounts of data from these different sources and making sense of it, enabling microgrids to operate more efficiently.
Real-time decision-making: Microgrids require real-time decision-making to ensure that energy is distributed efficiently and effectively. Machine learning algorithms are capable of making these decisions quickly and accurately, allowing microgrids to operate at optimal levels.
Optimization: Machine learning algorithms can optimize the operation of microgrids, ensuring that energy is produced, stored, and distributed in the most efficient and cost-effective manner. This can lead to significant cost savings and improved reliability.
Predictive maintenance: Machine learning algorithms can predict when equipment in a microgrid is likely to fail, allowing for proactive maintenance and reducing downtime.
Scalability: Microgrids can be scaled up or down depending on the energy needs of a particular location. Machine learning algorithms can adapt to changes in the size and complexity of a microgrid, ensuring that it operates efficiently regardless of its size.
If you don’t know what microgrids are then you must know that – Microgrids are localized energy systems that can operate independently or in conjunction with the main power grid, and they are increasingly being used to provide reliable and sustainable energy in remote or off-grid areas, as well as in urban settings.
Machine learning and AI can help microgrids to optimize their energy production, storage, and distribution in real time by analyzing large amounts of data from various sources such as weather patterns, energy demand, and energy supply. This enables microgrids to make data-driven decisions about how to allocate energy resources in the most efficient and cost-effective way possible, while also minimizing waste and reducing greenhouse gas emissions.
Some specific applications of machine learning and AI in microgrid energy management include:
- Predictive maintenance: Machine learning algorithms can be used to predict when equipment such as solar panels or batteries are likely to fail, allowing for proactive maintenance and reducing downtime.
- Load forecasting: AI algorithms can analyze historical energy usage data to predict future energy demand, enabling microgrids to adjust their energy production and distribution accordingly.
- Energy trading: Machine learning can help microgrids to optimize their energy trading strategies by analyzing market data and identifying the most profitable opportunities for buying and selling energy.
- Demand response: AI algorithms can be used to automatically adjust energy usage in response to changes in energy prices or grid conditions, helping microgrids to reduce their energy costs and improve grid stability.
Gridscape is using machine learning/ AI in many ways to optimize the operation of its microgrid technology. From energy forecasting to drawing insights, to better operation and maintenance, AI is helping Gridscape to run energy management of microgrids more efficiently by analyzing large amounts of data from various sources in real-time, enabling microgrids to make data-driven decisions about energy production, storage, and distribution. This results in the optimal use of renewable energy sources, reduced reliance on fossil fuels, and improved sustainability of the microgrid.
Overall, the use of machine learning and AI in microgrid energy management has the potential to revolutionize the way energy is produced, stored, and distributed, making energy systems more efficient, reliable, and sustainable.